RESEARCH ROUNDUP
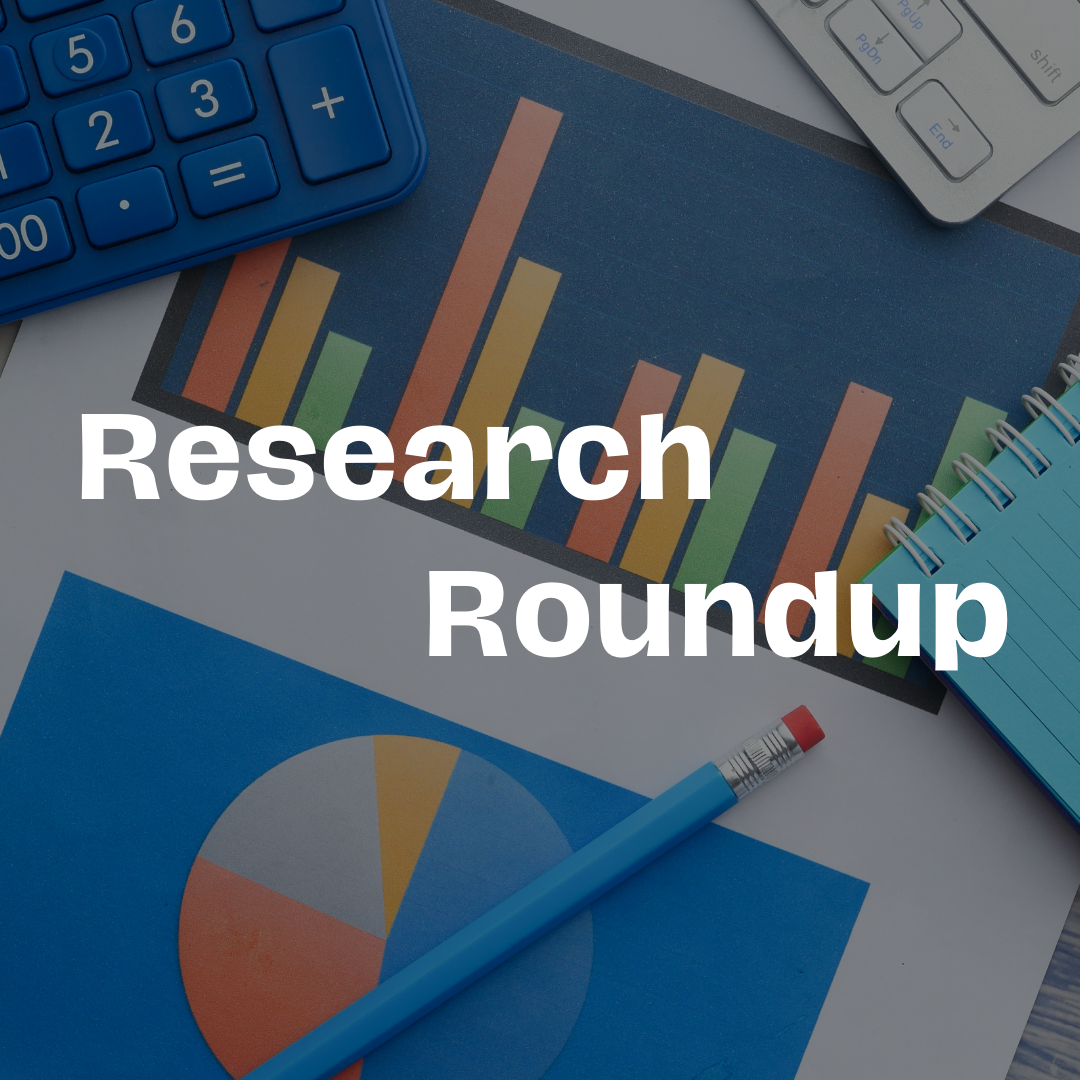
Title: Cloud computing infrastructure for “A gut-brain interaction controlling reward
learning”
Period: 09/23–05/24
Amount: $243,631
Role: Co-Investigator
Funding agency: National Institutes of Health
PI: Willam M. Howe (Virginia Tech)
Brief description: This award will support the development of a cloud-based
computational architecture to help identify the most efficient and affordable means of
managing the datasets that have become the bedrock of modern systems and
behavioral neuroscience, and provide a rubric for data management that can be applied
to multiple domains of experimental biology.
1)NIH NHLBI (grant number T32 HL079896): Integrated Biostatistical Training
for CVD Research (2022-2027). Role co-PD
This predoctoral training grant is in collaboration with Duke Biostatistics and Bioinformatics, and Duke Clinical Research Institute (DCRI) at Duke University. It aims to equip trainees for careers in biomedical research with a particular focus on cardiovascular diseases.
Source: FDA 1U01FD007934, 2023-2026
PIs: Xiaofei Wang and Shu Yang
Title: Methods to improve efficiency and robustness of clinical trials using information from real-world data with hidden bias
Description: North Carolina State University (NCSU, PI Shu Yang) in collaboration with Duke University (PI Xiaofei Wang) have been awarded up to $2.6 million by the U.S. Food and Drug Administration. The grant, spanning three years from September 1, 2023, to August 31, 2026, aims to innovate statistical methods that boost the efficiency and robustness of clinical trials. This is done by incorporating real-world data and taking into account hidden biases. This funding opportunity is a direct response to the FOA RFA-FD-23-025, which seeks to champion the application of Real-World Data in generating practical evidence for regulatory decision-making. The primary objectives of this FOA are to improve the quality and/or use of RWD, promote a better understanding of RWE study designs, and develop specific tools to evaluate aspects of RWE generation. Prominent contributors to this venture encompass Shein-Chung Chow, Ph.D., professor in the Duke Department of Biostatistics and Bioinformatics, Thomas Stinchcombe, MD, professor in the Duke Department of Medicine, Roee Gutman, associate professor in the Department of Statistics at Brown University, and Mingyang Shan, Doug Faries, and Kristin Sheffield of Eli Lilly & Company.
Army Research Office: Optimal Bayesian inference for high-dimensional, graphical and functional data. Proposal number 76643MA; 2020-0945.
Funding level $331,858. 05/01/2021–04/30/2024. Role: PI.
PI of an Alfred P. Sloan Foundation-funded book writing project (2023-2024) with the Libraries and Data Science Academy. They will produce a practical manual on how to provide data science consulting that can be followed at other institutions, as well as a white paper on the collaborative authoring of the manual.
NIH U24AR071113 Subcontract (2023). Molecular Transducers of Physical Activity Consortium Coordinating Center (MPI Miller, Pahor, Rejeski, Tracy). Role: Subcontract PI.
Description: Motivated by the belief that some wearable devices are better equipped at measuring some activities over others, we develop statistical models that are tailored to make recommendations about which wearable devices are best for specific activities and population groups.
Title: Copy Number Variation Identification and Association Study on Alzheimer’s Disease Whole Genome Sequencing Data
NIH RF1AG074328 (Lee/Tzeng)
Role: co-I
09/2021 – 08/2026
Goals: This study aims to identify copy number variations (CNVs) associated with Alzheimer’s Disease (AD) by analyzing whole genome sequencing data and to assess their contribution to AD risk using established and novel CNV association methods.
Title: Assisting Stroke Survivors with Engineering Technology – ASSET
Agency: NIDILRR (National Institute on Disability, Independent Living, and Rehabilitation Research)
Total Amount: $4.6M
Role: Co-I
Description (summarized from link): Despite rehabilitation efforts to promote recovery through therapy, the average stroke patient can experience persistent impairment of sensory and motor functions. The effects of stroke remain the leading cause of long-term disability in US adults. This project funds a Rehabilitation Engineering Research Center (RERC) in ASSET which aims to mitigate long-term effects through focused research and development on wearable assistive devices.
Other institutions/faculty: The center involves several members of Joint BME’s Closed Loop Engineering for Advanced Rehabilitation core (CLEAR), which include investigators from NC State’s College of Engineering, the Department of Statistics, the Department of Psychology and UNC-Chapel Hill’s School of Medicine.
Title: Novel Imprint Control Regions (ICRs) Responsive to Environmental Exposures
NIH R01ES032462 (Hoyo)
Role: co-I
09/2021-06/2026
Goals: The major goals of this project are to detect methylation marks that are responsive to environmental cadmium exposure and predictive of metabolic outcomes.
Statistical foundations for uncertainty quantification of machine intelligence assembled from gen- eralized fiducial inference (2023-2024). NC State Faculty Research and Professional Development Fund, sole-PI, 7,000 USD.
Seed grant: Hidden Markov methodology for assessing racial stressors (2023-2024). NC State Data Science Academy, PI, 50,000 USD
Source: 2023–2026 Patient-Centered Outcomes Research Institute ME-2022C2-25573
Title: Learning to Predict Disease Trajectories
PI: M.-S. Ong, Department of Population Sciences, Harvard Pilgrim
Description: The goal of this project is to develop a machine learning (ML) method to predict disease trajectories in patients with relapsing remitting diseases, with a focus on juvenile idiopathic arthritis. The team wants to learn if the method can help predict how likely it is for a patient to achieve an inactive disease state and identify patients who can achieve inactive disease without use of medications that can potentially have serious side effects. The team will also apply the method to study how different factors interact over time to affect disease trajectories. My role is to provide advice on statistical modeling and methodology for studying the sequential treatment decisions that are often involved.
- Categories: