Q2-2024 Research Roundup
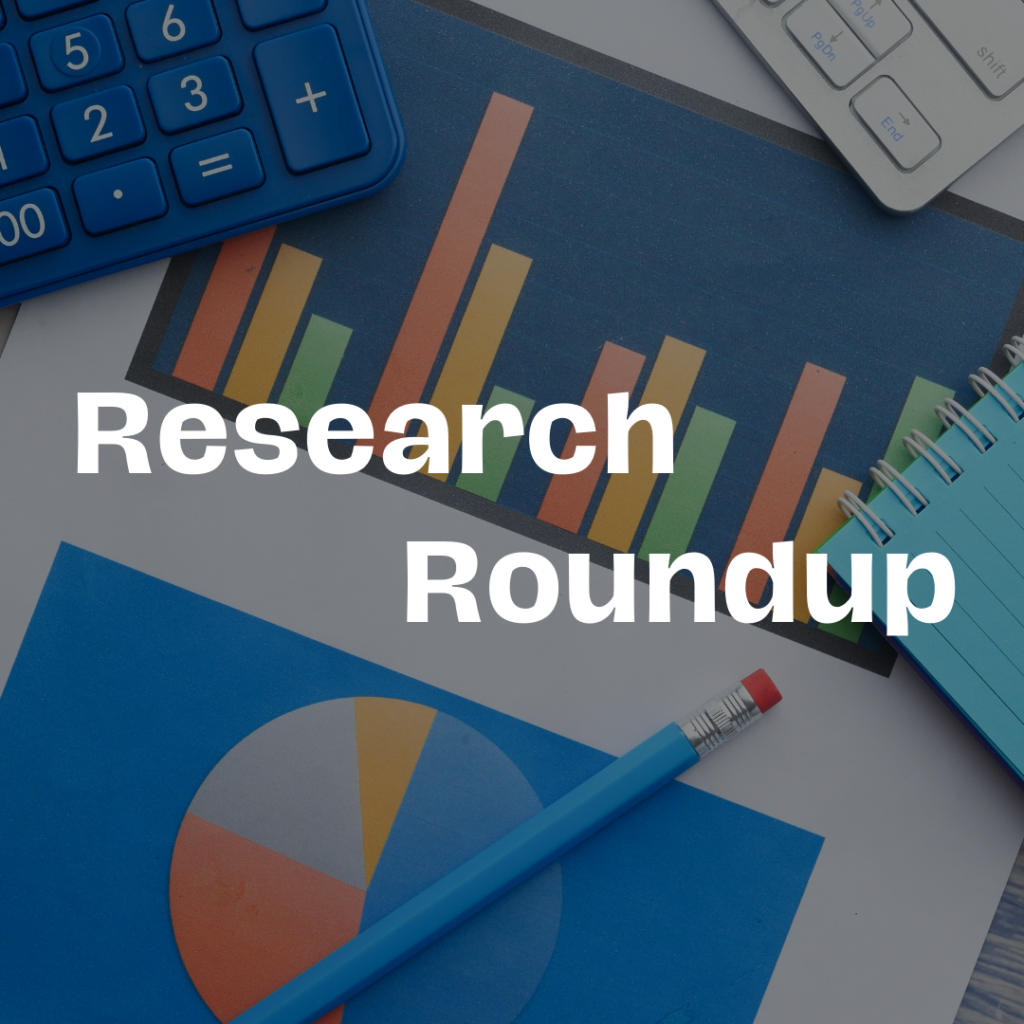
Source: National Institutes of Health R01 AG076577
Period: 2022–2027
Title: Sequential Multiple Assessment Randomized Trial of Exercise for PAD: SMART Exercise for PAD
PI: M. McDermott, Northwestern University
Description: Supervised exercise therapy (SET), consisting of treadmill exercise conducted 3 times/week at a center in the presence of healthcare personnel, is first line therapy for people disabled by lower extremity peripheral artery disease (PAD). However, traveling to a center for SET is burdensome. Home-based exercise is more accessible and less burdensome than SET. Yet, guidelines recommend SET over home-based exercise for PAD. The goal of this project is to conduct a sequential multiple assignment randomized trial (SMART) to study strategies for using SET and home-based exercise to optimize outcomes in patients with PAD. My role is to provide statistical leadership on the SMART design and analysis.
Source: NIH
Period: 2023-2030
Title: NC TraCS
Role: co-I
Description: Emily Griffith is a co-investigator on the NC State segment of the recently-renewed NC TraCS proposal (NIH, https://tracs.unc.edu/, 2023-2030)– in her role she will build and staff an interdisciplinary group of NC State students who will work with NC TraCS program. The students will work to support the data science needs of clinical researchers doing high-impact translational and collaborative work through workshops, online educational modules, and one-on-one consulting.
Source:NSF DMS 2152887
Period: (2022-2025).
Title: Projecting flood frequency curves under a changing climate using spatial extreme value analysis (PI Reich).
Role: co-PI.
Description: We develop new methodological and computational tools for spatial extreme value analysis and apply them to forecasting flood risk under a changing climate. The analysis combines fifty years of annual maximum streamflow observations at hundreds of gauges provided by the United States Geological Survey with CMIP6 climate model output produced under different shared socio-economic pathways.
Source: North Carolina State University Laboratory for Analytic Sciences
(National Security Agency)
Period: 01/24–12/24
Title: Statistical uncertainty quantification for large language knowledge graphs via
conformal prediction
Amount: $140,000
Role: Principal Investigator
co-PI: Dr. Karl Padzernik (Pacific Northwest National Laboratory)
Description: A knowledge graph (KG) is a graph-based representation of information about various entities (such as individuals, organizations, locations, or concepts) and their relationships. The transformation of unstructured language data into a structured KG facilitates downstream tasks like information retrieval, association mining, question answering, and machine reasoning. A celebrated example is the Google Knowledge Graph of 800 billion facts on 8 billion entities, from which Google serves relevant information in an infobox beside its search results. KG construction involves three steps: (i) Named Entity Recognition (NER), i.e., identifying the mention of named entities; (ii) Named Entity Disambiguation (NED), i.e., determining the identity of a named entity from context (e.g., “Jordan” could mean the country, the basketball player, or someone else), and (iii) Relationship Extraction (RE), i.e., determining the relationships among these entities. These steps typically employ black-box machine learning tools that do not produce statistically interpretable uncertainty quantification metrics, making it impossible to objectively assess how reliable the KG is. This project aims to develop statistically principled uncertainty quantification techniques for KGs by modeling how uncertainty propagates through the three steps. This will be accomplished by leveraging the proven statistical technique of conformal prediction.
Source: NCSU Seed Grant
Period: 2023-2024
Title: Modeling Social Media Information Pathways and Mitigating the Effect of Disinformation. Role: co-PI
Description: This project is centered on studying trends related to self-harm, harm towards others, and mental health issues as they are manifested on social media, and it also explores how COVID-19 has influenced these trends.
Source: NIH
Title: Toward Restoration of Normative Postural Control and Stability using Neural Control of Powered Prosthetic Ankles
Total Amount: $3.6M
Role: Co-I
Description: The objective of this proposal is to investigate the effects of a direct EMG-controlled powered prosthesis ankle on the transtibial amputee’s (Aim 1) balance and postural stability, (Aim 2) neuromuscular coordination mechanisms, and (Aim 3) cognitive function, compared to their daily prescribed devices. As lower-limb amputations cause altered residual muscle activity, and a powered prosthesis presents new limb dynamics, training residual muscle coordination for prosthesis operation in balance control is needed. Hence, we propose to provide a crafted prosthetics training and rehabilitation before evaluating either device (EMG-controlled powered ankle or daily passive prosthesis) in a crossover trial.
Other institutions/faculty: The project involves several members of Joint BME’s Closed Loop Engineering for Advanced Rehabilitation core (CLEAR), including investigators from NC State’s College of Engineering, the Department of Statistics, and the Department of Psychology.
Source: NIH RF1AG074328
Period: 09/2021 – 08/2026
Title: Copy Number Variation Identification and Association Study on Alzheimer’s Disease Whole Genome Sequencing Data
(Lee/Tzeng)
Role: MPI
Description: This study aims to identify copy number variations (CNVs) associated with Alzheimer’s Disease (AD) by analyzing whole genome sequencing data and to assess their contribution to AD risk using established and novel CNV association methods.
Source: NSF SES
Period: 2023-2026
Title: Causal Inference with Irregularly Spaced Observation Times
Role: PI
Description: Real-world data (e.g., consumer expenditures, mobile health applications, and electronic health records, EHRs) provide unique opportunities for discovering optimal treatment strategies for health care and society. However, complex data also present novel challenges for statistical analysis, such as time-dependent confounding, measurements at irregularly-spaced and informative observation times, mixed data types, which are impediments to effectively translating rich information into meaningful knowledge. The all-embracing theme of this proposal is to provide a causal inference and machine learning toolset to tackle these important and recurring challenges arising from emergent real-world data.
- Categories: